- Turing Post
- Posts
- FOD#55: AI Factories, New Industrial Revolution, and Look Back
FOD#55: AI Factories, New Industrial Revolution, and Look Back
+ cool news from the usual suspects and the best weekly curated list of research papers
Next Week in Turing Post:
Wednesday, AI 101: In the third episode, we discuss PEFT – Parameter-Efficient Fine-Tuning technique;
Friday: A new investigation into the world if AI Unicorns.
If you like Turing Post, consider becoming a paid subscriber. You’ll immediately get full access to all our articles, investigations, and tech series →
A new industrial revolution is unfolding, driven by the rise of AI factories. These facilities are changing computing across all scales, from massive data centers to everyday laptops, that very likely soon all turn into AI laptops. At Computex 2024, Jensen Huang highlighted this shift, emphasizing the need for industry-wide cooperation: meaning that hardware vendors, software developers, and enterprises have to make collective effort to transition from data centers to AI factories. Jensen Huang manifests that transformation is not just about technology but about reshaping the entire computing landscape. He usually knows what he says.
At the Nvidia pre-brief, the executives underlined the significant focus on the AI PC, a technology Nvidia introduced six years ago, in 2018. This innovation has revolutionized areas such as gaming, content creation, and software development.
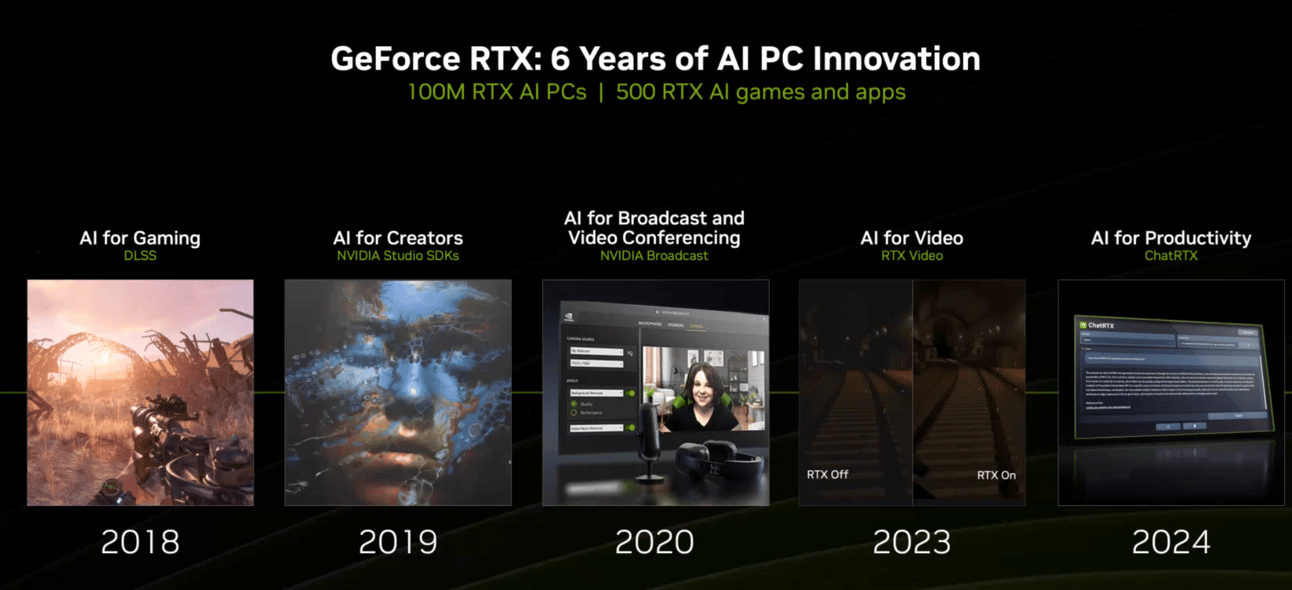
Image Credit: Nvidia
AI PCs were not something widely discussed over the last six years, but now –thanks to Microsoft and Nvidia – they are becoming ubiquitous. Along with conversations about the new industrial revolution. And while still at the threshold, it is indeed important to remember history. In 2018 and early 2019, another significant event sent shockwaves through the ML community. This event made possible the groundbreaking milestone: ChatGPT. Let’s walk through this timeline:
2018 the creation of GPT (generative pre-trained transformer) led to –>
Feb, 2019 GPT-2 – an LLM with 1.5 billion parameters. It was not fully released to the public, only a much smaller model was available for researchers to experiment with, as well as a technical paper, that led to –>
2020 GPT-3 and the paper Language Models are Few-Shot Learners, which evolved to –>
2022 GPT-3.5 and the fine-tuned version of GPT-3.5, called InstructGPT with the research paper Training language models to follow instructions with human feedback.
Nov, 2022 – ChatGPT. It was trained in a very similar way to InstructGPT, the magic behind it is based on the research paper Deep reinforcement learning from human preferences, the technique is called reinforcement learning from human feedback (RLHF).
Jack Clark, now a co-founder of Anthropic and formerly the Policy Director of OpenAI, reflected today on the launch of GPT-2, which he described as "a case of time travel." In 2019, OpenAI's decision to withhold the full release of GPT-2 due to concerns about misuse sparked a loud debate within the AI community. This debate centered on balancing innovation with ethical responsibility. Critics argued that withholding the model could slow scientific progress, while supporters applauded OpenAI's cautious approach.
Jack argues that departing from norms can trigger a counterreaction. By gradually releasing GPT-2, OpenAI unintentionally fueled interest in developing open-source GPT-2-grade systems, as others aimed to address the perceived gap. If GPT-2 had been fully released from the beginning, there might have been fewer replications, as fewer people would have felt compelled to prove OpenAI wrong.
There are many interesting questions in the Clark’s reminiscence of that turbulent times. It’s worth reading in full but here are a few quotes for your attention:
“Just because you can imagine something as being technically possible, you aren't likely to be able to correctly forecast the time by which it arrives nor its severity.”
"I've come to believe that in policy 'a little goes a long way' – it's far better to have a couple of ideas you think are robustly good in all futures and advocate for those than make a confident bet on ideas custom-designed for one specific future."
"We should be afraid of the power structures encoded by these regulatory ideas and we should likely treat them as dangerous things in themselves. I worry that the AI policy community that aligns with long-term visions of AI safety and AGI believes that because it assigns an extremely high probability to a future AGI destroying humanity, that this justifies any action in the present."
"Five years on, because of things like GPT-2, we're in the midst of a large-scale industrialization of the AI sector in response to the scaling up of these ideas. And there's a huge sense of deja vu – now, people (including me) are looking at models like Claude 3 or GPT-4 and making confident noises about the technological implications of these systems today and the implications of further scaling them up, and some are using these implications to justify the need for imposing increasingly strict policy regimes in the present. Are we making the same mistakes that were made five years ago?"
We don’t have an answer but can certainly make a few confident noises about this new industrial revolution, made possible due to scaling laws and now powered by AI factories. People like Jensen Huang argue that we are at the moment of redefining what is possible in technology. What do you think? To see the bigger picture of the future, we – as always – encourage you to know the past.
Additional reading: And even play with the past, like Andrej Karpathy did: he just released a method to train GPT-2 models quickly and cost-effectively. Training a tiny GPT-2 (124M parameters) takes 90 minutes and $20 using an 8xA100 GPU. The 350M version takes 14 hours and $200, while the full 1.6B model requires a week and $2.5k. The method uses Karpathy’s llm.c repository, which leverages pure C/CUDA for efficient LLM training without large frameworks.
Twitter Library
News from The Usual Suspects ©
The State of AI in Early 2024
According to McKinsey, the adoption of generative AI is spiking, beginning to generate substantial value.
OpenAI's: Threat Actors, Security Committee and Back to Robotics
OpenAI has released a detailed report highlighting the use of its AI models in covert influence operations by threat actors from Russia, China, Iran, and Israel. These operations, aimed at manipulating public opinion and political outcomes, were largely ineffective in engaging authentic audiences despite increased content generation. In response, OpenAI has implemented measures such as banning accounts, sharing threat indicators, and enhancing safety protocols. Notable campaigns include "Bad Grammar" (Russian), "Doppelganger" (Russian), "Spamouflage" (Chinese), "IUVM" (Iranian), and "Zero Zeno" (Israeli). This underscores the dual role of AI in both conducting and defending against covert IO, highlighting the necessity of a comprehensive defensive strategy.
They also established a Safety and Security Committee to address critical safety concerns. Led by Bret Taylor, Adam D’Angelo, Nicole Seligman, and Sam Altman, this committee is tasked with making safety recommendations within 90 days, supported by consultations with cybersecurity experts. These recommendations will be publicly shared to ensure robust safety and security measures across OpenAI’s projects.
In other news, OpenAI has relaunched its robotics team and is currently hiring. From the past (also 2018): Learning Dexterous In-Hand Manipulation. Back then, they developed a system called Dactyl, which is trained entirely in simulation but has proven to solve real-world tasks without physically-accurate modeling of the world.
Claude 3 Enhances Tool Integration
The Claude 3 model family now supports tool use, enabling interaction with external tools and APIs on Anthropic Messages API, Amazon Bedrock, and Google Cloud's Vertex AI.
NVIDIA's New AI Chip: Vera Rubin
At Computex, NVIDIA CEO Jensen Huang announced in his keynote the 'Vera Rubin' (named after American astronomer who discovered dark matter) AI chip, set to launch in 2026. This new chip will feature cutting-edge GPUs and CPUs designed for AI applications. NVIDIA plans to upgrade its AI accelerators annually, starting with the Blackwell Ultra in 2025, focusing on cost and energy efficiency.
They also introduced Earth Climate Digital Twin to be able to not only predict but know what’s happening with our planet and its climate.

Image Credit: Nvidia
Mistral AI Introduces Codestral
Researchers at Mistral AI have unveiled Codestral, a 22B open-weight generative AI model tailored for code generation. Supporting over 80 programming languages, Codestral excels in tasks like code completion and test writing, outperforming other models in long-range repository-level code completion. Available for research and testing via HuggingFace, Codestral also integrates with popular tools like VSCode and JetBrains, enhancing developer productivity.
Eleven Labs Introduces Text to Sound Effects (via video). Very impressive!
The freshest research papers, categorized for your convenience
Our top-3
French people know their decanting → Hugging Face introduces 🍷 FineWeb
In the blog post “🍷 FineWeb: decanting the web for the finest text data at scale,” researchers introduced a massive dataset of 15 trillion tokens derived from CommonCrawl snapshots. FineWeb, designed for LLM pretraining, emphasizes high-quality data through meticulous deduplication and filtering. They also developed FineWeb-Edu, a subset optimized for educational content, outperforming existing datasets on educational benchmarks. They were also able to make this blog post go viral! As always, kudos to Hugging Face for their light-hearted approach, care for open, transparent methods for creating large-scale, high-quality datasets for LLM training, and love of wine (but that’s an assumption).
Mamba-2 is here! Transformers are SSMs: Generalized Models and Efficient Algorithms Through Structured State Space Duality
Researchers from Princeton University and Carnegie Mellon University revealed that Transformers and state-space models (SSMs) share deep theoretical connections. They introduced a framework called structured state-space duality (SSD), showing that SSMs can be as efficient as Transformers. They designed Mamba-2, a refined SSM architecture, which is 2-8 times faster than its predecessor, Mamba (check here what Mamba is). Mamba-2 remains competitive with Transformers in language modeling tasks while optimizing memory and computational efficiency.
We’re just scratching the surface of these deep connections between attn and SSMs. What are these few attn layers doing in a hybrid model that improves quality? How do we optimize SSM training on new hardware (H100)? How does inference change if KV cache is no longer a thing?
9/— Tri Dao (@tri_dao)
3:24 PM • Jun 3, 2024
An Introduction to Vision Language Models (VLMs)
Researchers from a few notable institutions including Meta, Mila, MIT etc present an introduction to VLMs, explaining their functionality, training, and evaluation. They highlight the challenges in aligning vision and language, such as understanding spatial relationships and attributes. The paper categorizes VLMs into different families based on training methods like contrastive, masking, and generative techniques. It also discusses the use of pre-trained backbones to enhance model performance and explores extending VLMs to video data for improved temporal understanding.
AI Model Enhancements
Transformers Can Do Arithmetic with the Right Embeddings - Improves transformers' arithmetic abilities using novel embeddings →read the paper
Trans-LoRA: Towards Data-Free Transferable Parameter Efficient Finetuning - Enhances the transferability of parameter-efficient models without original data →read the paper
LOGAH: Predicting 774-Million-Parameter Transformers using Graph HyperNetworks with 1/100 Parameters - Utilizes graph networks to predict parameters efficiently for large models →read the paper
2BP: 2-Stage Backpropagation - Proposes a two-stage process for backpropagation to increase computational efficiency →read the paper
LLAMA-NAS: Efficient Neural Architecture Search for Large Language Models - Uses neural architecture search to find efficient model configurations →read the paper
VeLoRA: Memory Efficient Training using Rank-1 Sub-Token Projections - Reduces memory demands during training with innovative data projection techniques →read the paper
Zipper: A Multi-Tower Decoder Architecture for Fusing Modalities - Integrates different modalities efficiently in generative tasks using a multi-tower architecture →read the paper
NV-Embed: Improved Techniques for Training LLMs as Generalist Embedding Models - Advances embedding training for enhanced retrieval and classification tasks →read the paper
JINA CLIP: Your CLIP Model Is Also Your Text Retriever - Enhances CLIP models for text-image and text-text retrieval tasks using contrastive training →read the paper
AI Applications in Multimodal and Specialized Tasks
Matryoshka Multimodal Models - Enhances multimodal model efficiency using nested visual tokens →read the paper
Zamba: A Compact 7B SSM Hybrid Model - Combines state space and transformer models to create a compact, efficient hybrid model →read the paper
Similarity is Not All You Need: Endowing Retrieval-Augmented Generation with Multi-layered Thoughts - Integrates deeper contextual understanding in retrieval-augmented generation systems →read the paper
AI Ethics, Privacy, and Alignment
Offline Regularised Reinforcement Learning for LLM Alignment - Focuses on aligning AI behaviors with human intentions using reinforcement learning →read the paper
Value-Incentivized Preference Optimization: A Unified Approach to Online and Offline RLHF - Proposes a new approach to learning from human feedback that is applicable in both online and offline settings →read paper
Parrot: Efficient Serving of LLM-based Applications with Semantic Variable - Optimizes the serving of language model applications by leveraging application-level information →read the paper
The Crossroads of Innovation and Privacy: Private Synthetic Data for Generative AI - Explores techniques for maintaining privacy while using synthetic data in AI training →read the paper
Cognitive Capabilities of AI
LLMs achieve adult human performance on higher-order theory of mind tasks - Evaluates large language models' ability to perform complex cognitive tasks, comparing them to human performance →read the paper
Leave a review! |
Please send this newsletter to your colleagues if it can help them enhance their understanding of AI and stay ahead of the curve. You will get a 1-month subscription!
Thank you for reading! We appreciate you.
Reply